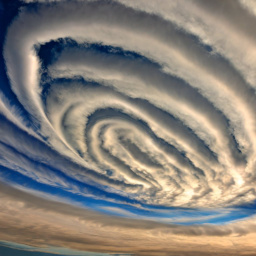
FedVEC
Client Selection in Federated Vehicular Clouds
Timeframe
25 November 2022 - 31 March 2024Extension
An extension from 31 October 2023 to 31 March 2024, together with additional funds, was granted by FAPESP.
Institutions
- TU Dresden
- University of Campinas
Team @ TU Dresden
Funding
- FAPESP
Description
Due to the increased computational capacity of Connected and Autonomous Vehicles (CAVs) and worries about transferring private information, it is becoming more and more appealing to store data locally and move network computing to the edge. This trend also extends to Machine Learning (ML) where Federated learning (FL) has emerged as an attractive solution for preserving privacy. Today, to evaluate the implemented vehicular FL mechanisms for ML training, researchers often disregard the impact of CAV mobility, network topology dynamics, or communication patterns, all of which have a large impact on the final system performance. As a first step towards addressing this, investigations of client selection in FL for VEC are needed.
Selected Publications
-
Wellington Viana Lobato Junior, Joahannes B. D. da Costa, Allan Mariano de Souza, Denis Rosário, Christoph Sommer and Leandro Aparecido Villas, "FLEXE: Investigating Federated Learning in Connected Autonomous Vehicle Simulations," Proceedings of 96th IEEE Vehicular Technology Conference (VTC 2022-Fall), London, United Kingdom, September 2022. [DOI, BibTeX, PDF and Details...]